Skin Color Detection Using Neural Networks
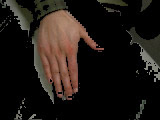
Another cool project I did back in college was to create a classifier that could look at a digital photograph or video and discern where there was human skin. Once you know where the skin is, you can analyze the shape of it, or treat it like a lamina and find its "center of mass" and how it changes over time in a video. However, before you can start thinking about scanning for people and interacting with their motion (or whatever your application may be), you must first learn how to collect and interpret the raw data. Skin in an image is found by analyzing the colors. Digital images exist in a "color space" which is a mapping of each distinct color to a particular point on a graph whose axes relate to very basic properties of colors. The most common color space is RGB -- each color can be defined by the percentage of red, green, and blue it contains. (Well actually, there are some colors that can't be represented in the RGB space, but most cameras can...